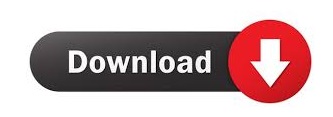

Heteroscedasticity (sometimes spelled as heteroskedasticity) occurs when the reading of a variable’s standard error (SE) measured over a given time is not constant.Īny regression analysis running on such data that exhibits heteroscedasticity gives, at the very least, biased coefficients and ruin the results. If the multicollinearity goes too high, it causes disturbance of data and the model falls apart. It is crucial to find out how input variables relate to each other.Measuring the multicollinearity of the regression model is a way to find the relationship between input variables.As an instance, you may come across a model in which you are willing to find out what determines the salary of a person at a particular of age.Independent variables (factors) such as educational background, age, and many other factors that influences average salary of an individual are brought under consideration.īut, before you go any further and throw every factor under the Sun in your model, you need to know how they correlate (inter-associate). In regression analysis, looking at the correlation between two or more input variables, it is observable that when the addition of one or more input variables takes place, the model fails to make things more transparent about the real world. Suddenly, Bill Gates and Jeff Bezos step into the building, and once you include the salaries of these two billionaires, the mean salary becomes drastically inaccurate.The salaries of these two well-known gentlemen are the outliers in this example. They all have a mean salary of around one hundred thousand dollars a year. The outlier points are essential because they can heavily influence the outcome of a regression analysis.To understand this concept, let’s suppose that a building is filled with professionals with average financial backgrounds in terms of their earnings. In regression analysis( 1), the outliers are points on the graph that fall significantly outside the cloud made up of other points. Outliers are visible with data plots on a graph. If the beta coefficient is negative, the interpretation is that for every 1-unit increase in the predictor variable, the outcome variable will decrease by the beta coefficient value.To understand types of regression analysis, understanding the related terminologies is useful. How do you interpret a negative beta in regression? If X never equals 0, then the intercept has no intrinsic meaning. If X sometimes equals 0, the intercept is simply the expected mean value of Y at that value. Start with a regression equation with one predictor, X. The intercept (often labeled the constant) is the expected mean value of Y when all X=0.

The negative intercept tells you where the linear model predicts revenue (y) would be when subs (x) is 0.Īlso question is, how do you interpret a regression intercept? The slope coefficient means something like that (but different to it). If you extend the regression line downwards until you reach the point where it crosses the y-axis, you'll find that the y- intercept value is negative! In fact, the regression equation shows us that the negative intercept is -114.3.įurthermore, can an intercept be negative? The negative intercept does not mean "that one sub increase would mean a revenue increase of 24.4".
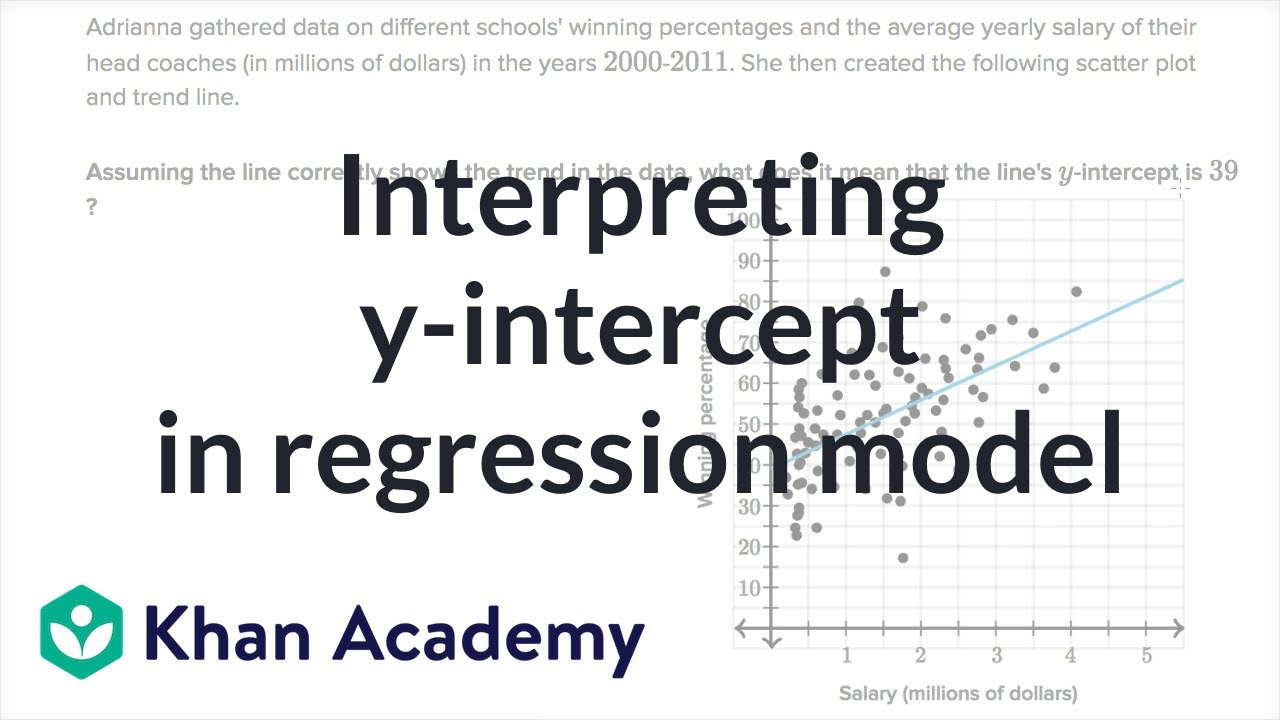
Likewise, how do you interpret a negative y intercept? This simply means that the expected value on your dependent variable will be less than 0 when all independent/predictor variables are set to 0. Depending on your dependent/outcome variable, a negative value for your constant/ intercept should not be a cause for concern.
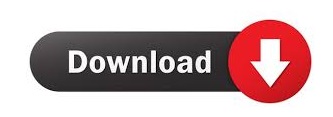